Fourth Wing Annotation Guide: Your Ultimate Resource For Expert Annotations
Apr 02 2025
Fourth wing annotation has become an increasingly popular topic in the world of data science, machine learning, and artificial intelligence. As data continues to grow exponentially, the need for accurate and efficient annotation tools becomes more critical than ever. Whether you're a beginner or a seasoned professional, understanding the fourth wing annotation guide can significantly enhance your data labeling processes and improve model performance.
This guide is designed to provide you with a comprehensive understanding of fourth wing annotation, its importance, and how it can benefit your projects. Whether you're working on image recognition, natural language processing, or other machine learning applications, mastering the art of annotation is essential for success.
Throughout this article, we will explore the various aspects of fourth wing annotation, including its methodologies, tools, best practices, and industry standards. By the end of this guide, you will have a solid foundation to implement effective annotation strategies in your own projects.
Read also:Unlock Your Creativity With The Trendy Angry Dog Filter
Table of Contents
- Introduction to Fourth Wing Annotation
- The Importance of Fourth Wing Annotation
- Fourth Wing Annotation Methodologies
- Tools for Fourth Wing Annotation
- Best Practices for Fourth Wing Annotation
- Common Challenges in Fourth Wing Annotation
- Applications of Fourth Wing Annotation
- Statistics and Industry Trends
- The Future of Fourth Wing Annotation
- Conclusion and Call to Action
Introduction to Fourth Wing Annotation
What is Fourth Wing Annotation?
Fourth wing annotation refers to the process of labeling or tagging data in a way that enhances its usability for machine learning and artificial intelligence models. This technique is particularly useful in scenarios where large datasets need to be processed and analyzed efficiently. By providing clear and accurate annotations, data scientists can train models that perform better and deliver more accurate results.
Fourth wing annotation involves assigning meaningful labels to data points, such as images, text, or audio files. These labels help machines understand the context and meaning behind the data, enabling them to make informed decisions. The quality of annotation directly impacts the performance of machine learning models, making it a critical component of the data preparation process.
Why is Fourth Wing Annotation Important?
In today's data-driven world, the ability to process and analyze vast amounts of information is crucial for businesses and organizations. Fourth wing annotation plays a vital role in this process by ensuring that data is properly labeled and categorized. This, in turn, allows machine learning models to learn from the data and make accurate predictions or classifications.
The Importance of Fourth Wing Annotation
Enhancing Model Accuracy
One of the primary benefits of fourth wing annotation is its ability to enhance the accuracy of machine learning models. By providing high-quality annotations, data scientists can train models that are better equipped to handle complex tasks, such as image recognition, speech processing, and natural language understanding.
Improving Data Usability
Another significant advantage of fourth wing annotation is its ability to improve the usability of data. Properly labeled data is easier to analyze and interpret, making it more valuable for businesses and organizations. This, in turn, leads to better decision-making and more efficient operations.
Fourth Wing Annotation Methodologies
Supervised Learning
Supervised learning is one of the most common methodologies used in fourth wing annotation. This approach involves training machine learning models using labeled datasets, where the input data is paired with the correct output. By using this method, models can learn to recognize patterns and make accurate predictions based on the provided annotations.
Read also:Gigi Salazar Michigan Unveiling The Inspiring Journey Of A Rising Star
Unsupervised Learning
In contrast to supervised learning, unsupervised learning does not rely on labeled datasets. Instead, it focuses on identifying patterns and structures within the data itself. While this approach can be useful in certain scenarios, it often requires additional annotation techniques to achieve optimal results.
Semi-Supervised Learning
Semi-supervised learning combines elements of both supervised and unsupervised learning. This methodology uses a small amount of labeled data along with a larger set of unlabeled data to train machine learning models. By leveraging the strengths of both approaches, semi-supervised learning can achieve high levels of accuracy while minimizing the need for extensive annotation efforts.
Tools for Fourth Wing Annotation
Popular Annotation Tools
There are several popular tools available for fourth wing annotation, each with its own unique features and capabilities. Some of the most widely used tools include:
- Labelbox
- SuperAnnotate
- V7
- CVAT
Selecting the Right Tool
When choosing a tool for fourth wing annotation, it's important to consider factors such as ease of use, scalability, and compatibility with your existing workflows. Additionally, you should evaluate the cost and support options offered by each tool to ensure that it meets your specific needs and budget constraints.
Best Practices for Fourth Wing Annotation
Defining Clear Guidelines
One of the key best practices for fourth wing annotation is establishing clear guidelines for annotators. By providing detailed instructions and examples, you can ensure that annotations are consistent and accurate across all datasets. This, in turn, leads to better model performance and more reliable results.
Using Quality Control Measures
Implementing quality control measures is another important best practice for fourth wing annotation. This can include regular audits of annotated data, peer reviews, and automated checks to ensure that annotations meet the required standards. By maintaining high levels of quality, you can improve the overall effectiveness of your machine learning models.
Common Challenges in Fourth Wing Annotation
Data Complexity
One of the primary challenges in fourth wing annotation is dealing with complex datasets. These datasets may contain ambiguous or incomplete information, making it difficult to assign accurate labels. To overcome this challenge, it's important to develop robust annotation strategies that can handle a wide range of data types and formats.
Scalability
Another common challenge in fourth wing annotation is scaling annotation efforts to meet the demands of large datasets. This can be particularly challenging for organizations with limited resources or expertise in data annotation. By leveraging advanced tools and techniques, you can streamline the annotation process and achieve greater efficiency.
Applications of Fourth Wing Annotation
Image Recognition
Fourth wing annotation is widely used in image recognition applications, where it helps machines understand and interpret visual data. By providing accurate annotations, data scientists can train models that are capable of recognizing objects, people, and scenes with high levels of precision.
Natural Language Processing
Another important application of fourth wing annotation is in the field of natural language processing (NLP). Here, annotations are used to label text data, enabling machines to understand and process human language more effectively. This has numerous applications, including chatbots, virtual assistants, and sentiment analysis tools.
Statistics and Industry Trends
According to recent studies, the global market for data annotation is expected to grow significantly over the next decade, driven by increasing demand for machine learning and artificial intelligence solutions. In fact, the market is projected to reach $3.5 billion by 2025, with a compound annual growth rate (CAGR) of 25%.
As more businesses and organizations adopt machine learning technologies, the need for high-quality annotation tools and services will continue to rise. This presents a significant opportunity for companies that specialize in fourth wing annotation to expand their offerings and capture a larger share of the market.
The Future of Fourth Wing Annotation
Emerging Technologies
Looking ahead, several emerging technologies are expected to have a major impact on the field of fourth wing annotation. These include advancements in computer vision, natural language processing, and automated annotation tools. As these technologies continue to evolve, they will enable more efficient and accurate annotation processes, further enhancing the capabilities of machine learning models.
Increased Automation
Another trend to watch in the future of fourth wing annotation is the increasing use of automation. By leveraging machine learning algorithms and artificial intelligence, organizations can automate many aspects of the annotation process, reducing the need for manual intervention and improving overall efficiency.
Conclusion and Call to Action
In conclusion, fourth wing annotation is a critical component of modern data science and machine learning workflows. By understanding the methodologies, tools, and best practices associated with this technique, you can enhance the accuracy and effectiveness of your models while improving data usability. As the field continues to evolve, staying up-to-date with the latest trends and technologies will be essential for success.
We encourage you to explore the resources and tools mentioned in this guide and apply them to your own projects. Additionally, we invite you to share your thoughts and experiences in the comments section below. Your feedback and insights can help others in the community learn and grow. Don't forget to check out our other articles for more valuable information on data science and machine learning topics.

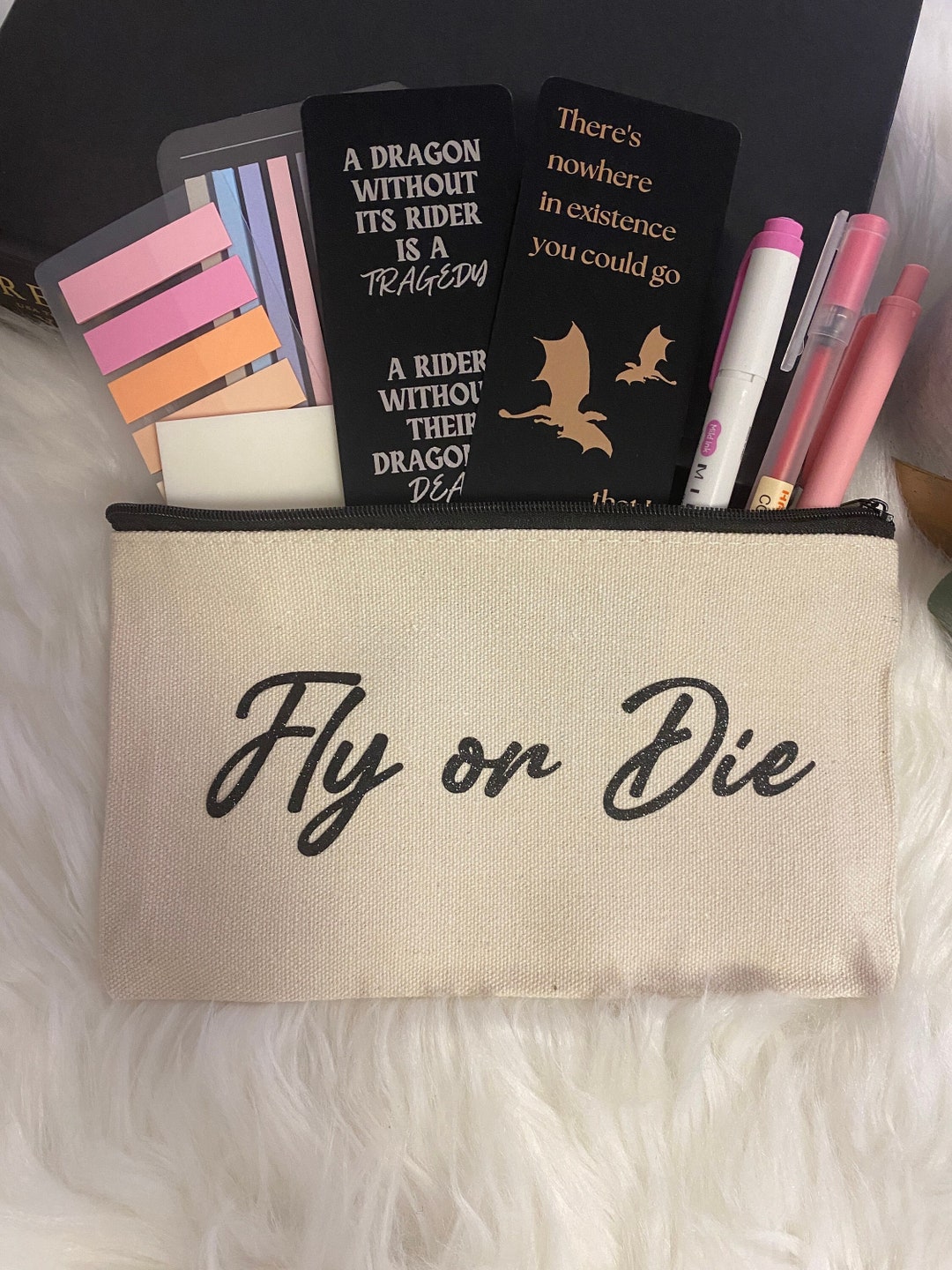
